Recently, the 2024 SIGMOD International Conference on Management of Data was held in Santiago, Chile. The ACM SIGMOD Committee on Data Management awarded the Systems Award to the development team of the Apache SINGA project, recognizing their contributions in 'developing a distributed, efficient, scalable, and user-friendly deep learning platform for large-scale data analytics.' Associate Professor Wu Yuncheng from the Laboratory of Data Warehousing and Business Intelligence at the School of Information was honored as a member of the Apache SINGA project development team, marking the first time a faculty member from our university has received this award.
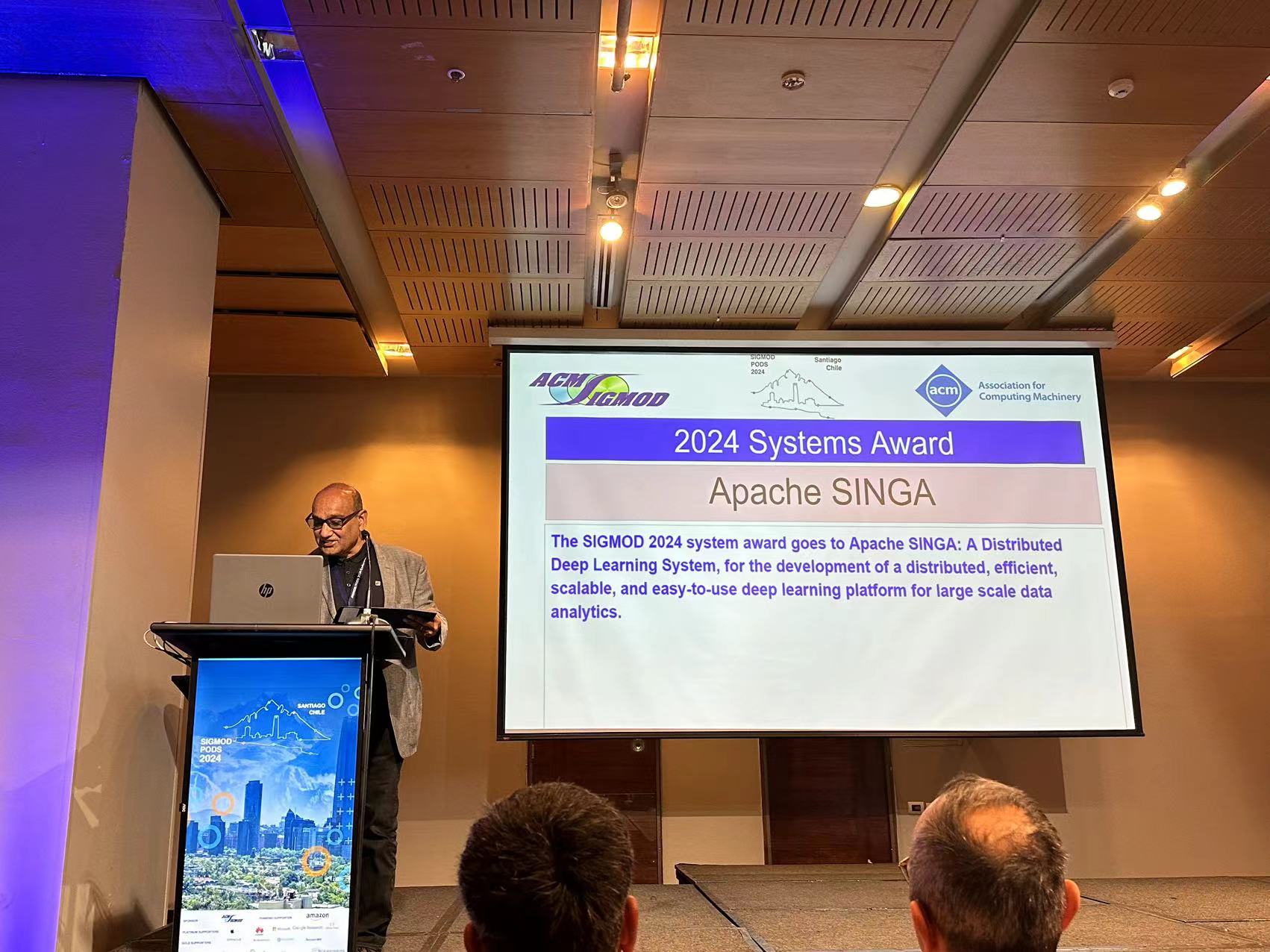
The ACM SIGMOD Systems Award is a premier honor in the field of databases, recognizing individuals or organizations that have made outstanding contributions to the theory and practice of large-scale data management systems. Past recipients include individuals or development teams behind renowned databases and data management systems such as Apache Flink, SQLite, and Postgres. For information on the 2024 award recipients, please visit the following link: https://sigmod.org/sigmod-awards/sigmod-systems-award/
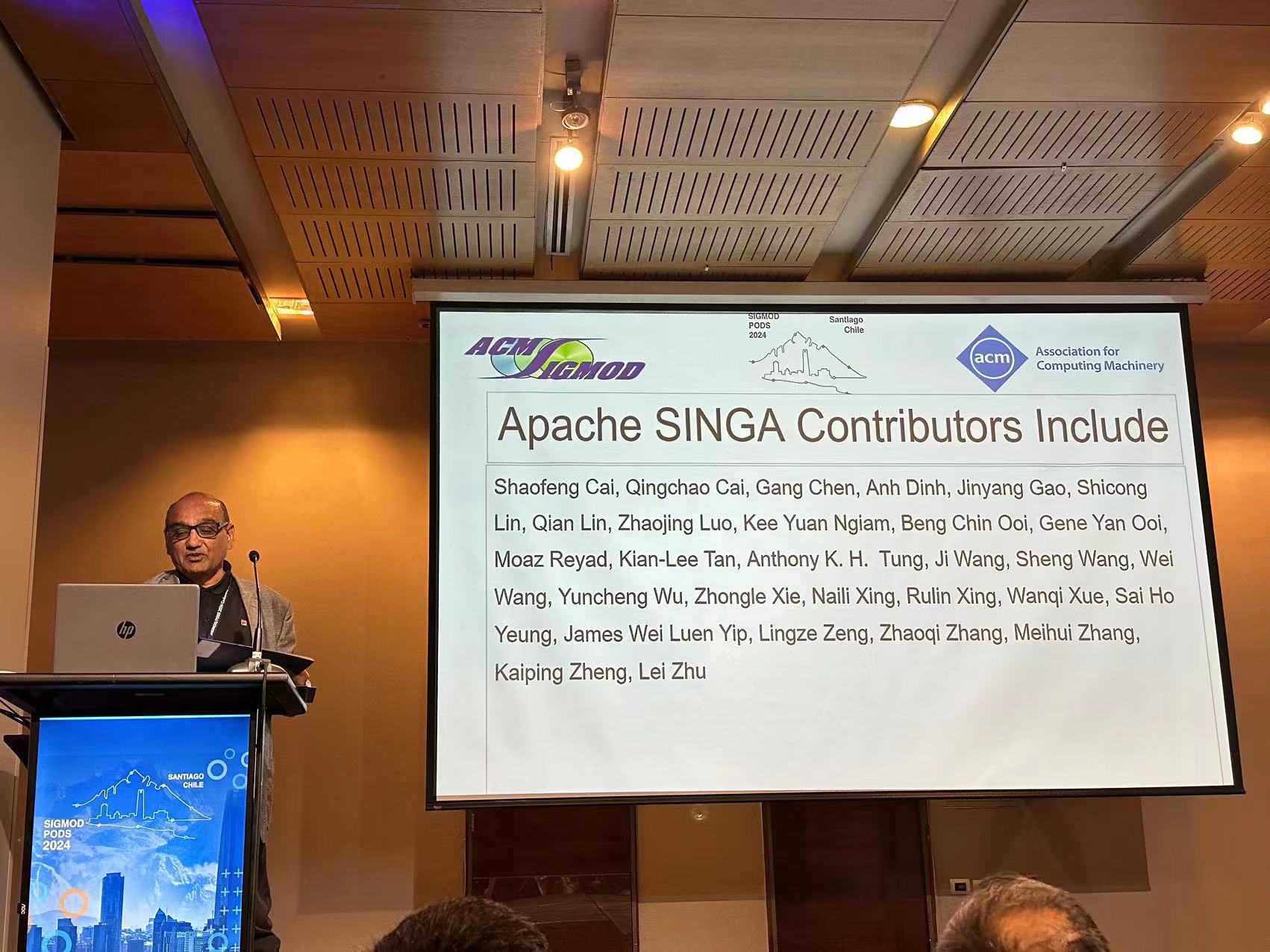
The Apache SINGA project was initiated in 2014 by Professor Ooi Beng Chin, a foreign member of the Chinese Academy of Sciences, fellow of the Singapore Academy of Sciences, fellow of the Singapore Academy of Engineering, and professor at the National University of Singapore. It was a joint effort with Zhejiang University and NetEase, incubated under the Apache Software Foundation as the first open-source deep learning platform. In 2019, it was promoted to become a top-level Apache project.
From 2019 to 2023, Associate Professor Wu Yuncheng was deeply involved in the project during his tenure at the National University of Singapore. Over the past decade, the Apache SINGA project has released four major versions, focusing on flexible training frameworks, system usability, novel programming models, and database-integrated analytics stages. Core components such as the Rafiki deep learning model training and inference module, the PANDA AI-as-a-service module, and the MLCask machine learning pipeline management module have been successively introduced.
Since 2023, the project team has concentrated on providing AI-based database analytics extensions for relational database management systems like PostgreSQL and PolarDB. In the latest v4.x release, Apache SINGA offers a model selection module seamlessly integrated into RDBMS, supporting SQL-aware model slicing for more accurate predictions of dynamic subsets of database records. The team continues to explore the intersection of AI and databases, striving for technological integration and innovation.
Additionally, the project team is committed to providing high-quality data for AI model training and ensuring data security and privacy during data flow processes. Apache SINGA has been applied in various fields including healthcare and financial analysis, with users such as Alibaba Cloud, NetEase, Singapore National University Hospital, and Siemens. For more information about Apache SINGA, visit the official website at https://singa.apache.org/.
Introduction
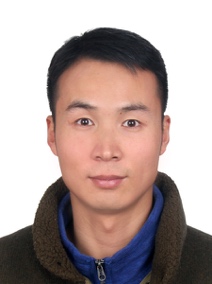
Wu Yuncheng is an associate professor at the School of Information of Renmin University of China. He previously served as a postdoctoral researcher and research assistant professor at the National University of Singapore. His primary research interests include data privacy and security, federated learning, and serverless computing. He has published over 30 papers in renowned academic journals and conferences such as SIGMOD, VLDB, ICDE, and TKDE. He has been awarded the SIGMOD 2024 Systems Award and nominated for the Best Paper Award at ICDE 2024.
Wu Yuncheng has also served as co-chair for the Demo Track of the international conference VLDB 2024 and as co-chair for the Workshop at BigComp 2023.
As a team member, Wu Yuncheng contributed to the development of the privacy-preserving federated learning system Falcon. This system addresses data security and privacy leakage issues faced by multiple parties in data collaboration. Falcon proposes a federated data collaboration framework and efficient protocols based on technologies such as homomorphic encryption, secure multiparty computation, zero-knowledge proofs, and differential privacy. It supports the training and inference of various models in horizontal and vertical federated scenarios. The project is open-source on GitHub and is in the stage of achieving practical results, aiming to promote the secure circulation of data elements and their value.